New Preprint Available
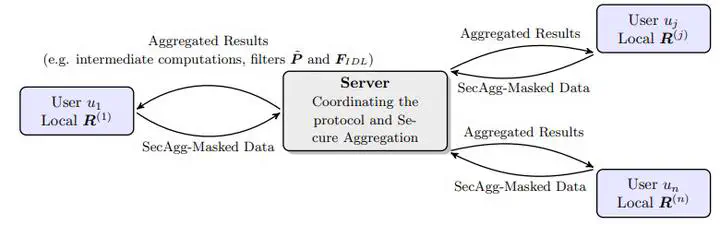
Secure Federated Graph-Filtering for Recommender Systems
Recommender systems rely on graph-based filters, but their centralized computation poses privacy and security risks. Our work introduces two decentralized frameworks that securely compute key graph components without centralizing user data. Leveraging Multi-Party Computation and distributed spectral methods, we ensure privacy while maintaining predictive accuracy. Our second approach further optimizes efficiency through low-rank approximations, balancing performance and communication costs. Experiments on benchmark datasets show that our methods match state-of-the-art accuracy while preserving data confidentiality. This work paves the way for privacy-preserving, decentralized recommender architectures.
-
You can already read this publication on arxiv: Secure Federated Graph-Filtering for Recommender Systems
-
Scientific Publications: Stay updated with the latest research outputs from our team, published in top-tier conferences and journals. Publications